Designs of the Times or, a Modern American Fable
How Can My Yield be Increased?
An experienced plant researcher was invited into the president’s office.Mr. President: “Come in, Dr. Researcher. I have an assignment for you. The manufacturing costs for our product POLYGLOP 123 are too high. I want you to conduct a statistically designed experiment to optimize the process and increase the yield.”
Dr. Researcher says, “I’ll get on it right away. I’ll conduct a process design study.”
The Steps in a Statistically Designed Experiment
Dr. Researcher should plan for five steps in conducting his statistically designed experiment.First, he should gather information to decide on what variables and variable range should be studied.
Second, he should decide on the experiments to be run and test the extremes of the test range to ensure success. If the test range is too narrow, Dr. Researcher should know that he might miss a new opportunity. But if the test range is too wide, several experiments may fail and invalidate the design. If all the extreme experiments are successful, Dr. Researcher may broaden the limits, but if the extremes fail, he would have to reduce the limits.
Third, Dr. Researcher would define the resources so that he knows how much money, time, staff, etc., is available. The number of experiments, the number of replicates and the experiments to be replicated are chosen.
Test methods are selected on the basis of applicability, accuracy and precision.
After considering these factors, the next step would be to conduct the experiments and analyze the results in order to either determine the optimum conditions or decide on the next round of experiments.

How Did He Proceed to Optimize His Process?
Dr. Researcher was aware that this was an established manufacturing process, with established quality-control methods, so he knew that the tests had good accuracy and precision. The process was currently being run at 160ºC for 4 hours. Dr. Researcher knew that the temperature of the reaction mixture and the length of the reaction time affected product yield, and decided to quantify their effects. For the first set of experiments, he decided to hold the time constant and vary the reaction temperature. The results of his initial set of experiments are plotted in Figure 1.
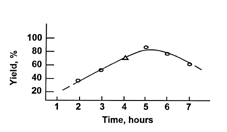
Dr. Researcher looked at his new results and was even more excited. “My goodness! My second design identified conditions to increase the yield to 85%, an increase of greater than 45% from the current process. Now all I have to do is refine this a bit,” he says.
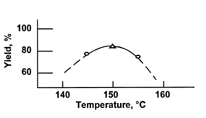
Dr. Researcher looked at his latest results and was disappointed to see no increases.
He thought, “The last experiment I have to do is to make sure that I haven’t missed anything.”
In his final design, Dr. Researcher held the reaction temperature constant at 150ºC and varied the time in half-hour increments. He did this to fill in the points at the top of the hill in Figure 2. The final results, shown in Figure 4, showed no additional improvement.
Dr. Researcher was very pleased with his accomplishment. Not only did he increase the yield of POLYGLOP 123 from 58% to 85%, he also identified the conditions that give the maximum yield. Very proudly he completed his report and waited in anticipation for his next meeting with Mr. President.
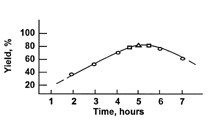
Mr. President’s Reaction to the Good News
Mr. President asks, “Well, Dr. Researcher, what excuses do you have for me?”“What?!” Dr. Researcher was dumbfounded.
“You reported that you increased the yield of POLYGLOP 123 to 85%,” Mr. President says. “Today I had lunch with my chief competitor for POLYGLOP 123 and, I hate to admit, bragged a little about your findings. My competitor laughed and said his yield is greater than 90%, and hinted that it was substantially over 90%.
“Go back and finish your work!”

What Did Dr. Researcher Do Wrong?
When research is done on a one-variable-at-a-time basis, like Dr. Researcher did, it is possible to leave vast regions of experimental space unexplored. Let’s look at the experiment a different way.Figure 5 depicts both temperature and time variables at the same time. Included are the experiments that Dr. Researcher completed.
The figure shows that doing a traditional one-variable-at-a-time study misses much experimental space. Dr. Researcher shouldn’t be blamed for his oversight; this approach isn’t unusual. In fact, it was what this author was taught when I went to school and first started working in industry. Fortunately, I was befriended by a chemical engineer who set me straight. It is recommended that every student or researcher take a course on applied statistics.1 There are many available — from colleges, private corporations and even the American Chemical Society.

The Correct Approach to Experimental Design
Instead of conducting a one-variable-at-a-time study, an orthogonal study is suggested. Orthogonal means running experiments where both variables are changed during the series, but are changed independently of one another. For example, two variables, such as time and temperature, are run at low, low; low, high; high, low; and high, high levels, respectively. The best experimental screening design for two ingredients is a “square.” A center point experiment in the middle of the design is optional (see Figure 6), but it is a good idea to include one or more of these so that an estimate of curvature can be made. In addition, if the center point is replicated, the experimental error can be calculated.# Figure 6 shows the experimental points Dr. Researcher settled on after he took a course on experimental design. The graph shows the conditions he chose to evaluate: ± 2 hours and ± 20ºC from the current process being run at 160ºC for 4 hours.
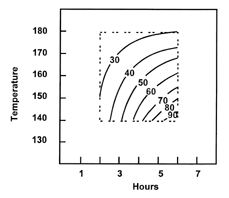
Figure 7 is a topographical map of the yield and shows that the yield could be raised from 58% to 90+%. One might be tempted to stop here, but Dr. Researcher wanted to be able to report the exact conditions for maximum yield to Mr. President.

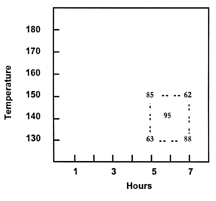
In this case, a response surface could not be generated, since not enough experiments of the correct type were present in the design. An ANOVA showed that second order terms were present, but couldn’t be defined because, again, not enough experiments of the correct type were present in the design. It certainly appeared to Dr. Researcher that there was a maximum in there someplace, but the experimental error prevented him from deciding if there was a ridge or a maximum. Dr. Researcher decided that further experimentation would be needed to completely define the topographical map.
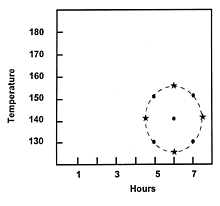
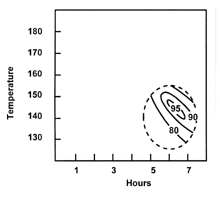
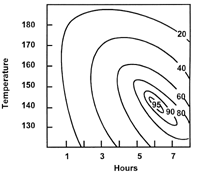
In later years, Dr. Researcher had time to define the response surface for the whole region (see Figure 12). He was very proud that his statistically designed experimentation uncovered the maximum yield possible.
Armed with data tables and graphs, Dr. Researcher scheduled another meeting with Mr. President. Dr. Researcher’s report documented two conclusions, 1) A 67% increase in yield of POLYGLOP 123 from 58% to 97% was achieved by changing the manufacturing conditions from 4 hours at 160ºC to 6 hours and 142ºC; and 2) 97% is an absolute maximum yield for this process. This time, when Dr. Researcher sent his report to Mr. President, he did so with confidence.
What Was Mr. President’s Reaction to the Second Report?
Mr. President, “Congratulations, Dr. Researcher! You have saved the company! You are hereby promoted to vice president of Research and Manufacturing.”Looking for a reprint of this article?
From high-res PDFs to custom plaques, order your copy today!