Achieving Six Sigma Objectives for Variability Reduction in Coating Formulation and Processing

Applying design of experiments (DOE) tools can make your coatings system more robust to variations in component levels and processing factors. This is the third article in a series on DOE.1,2
"Six sigma" is the new rallying cry for quality improvement in the chemical process industry. For example, Dow aims to generate an extra $1.5 billion per year in profits after training 50,000 of its employees on the methods of six sigma.3 Statistical tools play a key role in achieving savings of this magnitude. In fact, "sigma" is a Greek letter that statisticians use as a symbol for standard deviation -- a measure of variability. If a manufacturer achieves a six-sigma buffer from its nearest specification, they will experience only 3.4 off-grades per million lots. This translates to better than 99.99966% of product being in specification. To illustrate what this level of performance entails, imagine playing 100 rounds of golf a year: At six sigma you'd miss only 1 putt every 163 years!4
Of all the statistical tools used within six sigma, design of experiments (DOE) offers the most power for making breakthroughs. By way of a case-study example, this article demonstrates how DOE can be applied to development of a coatings system to achieve optimal performance with minimum variability, thus meeting the objectives of six sigma programs.

Minimizing Propagation of Error (POE) from Varying Inputs
After earning his Ph.D. in chemistry and taking a job at a chemical company, a colleague of ours got assigned to an operator for an orientation to the real-world of production. As the operator watched with much amusement and disgust, the chemist carefully weighed out materials with a small scoop. The operator pushed the Ph.D. chemist aside, grabbed a sack of chemicals and tossed it into the reactor. "You're not in the laboratory anymore," he said, "This is how we do things in manufacturing."
Hopefully the operators of your coatings systems will be more exacting when adding ingredients. However, at the very least, you can expect some variation due to inherent limitations in equipment. How will these variations affect product quality and process efficiency? Can you do anything to make your system more robust to variations in component levels and process settings? The answers to these questions can be supplied by way of an advanced form of DOE called "response surface methods" (RSM). This statistical tool produces maps of product and product performance, similar to topographical displays of elevation, as a function of the input variables that you (or your clients) control. The objective of six sigma is to "find the flats" -- the high plateaus of product quality and process efficiency that do not get affected much by variations in component levels or factor settings. You can find these desirable operating regions visually, by looking over the 3-D renderings of response surfaces, or more precisely by way of a mathematical procedure called "propagation of error" (POE).
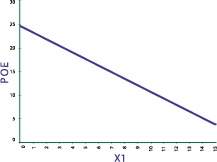
Assume that this factor exhibits a constant variation shown on the graph as a difference with magnitude delta (D). This variation, or error, will be transmitted to the measured response to differing degrees depending on the shape of the curve at any particular setting. In this example, because the curve flattens out as the control factor increases, a setting at the higher level causes less POE. Therefore, you see a narrower difference (DY) on the response axis as a result of setting the factor at the higher, rather than lower, level.
With the aid of some calculus, the POE itself can be graphed as a continuous function. In this case the original response surface can be described by the following quadratic equation.
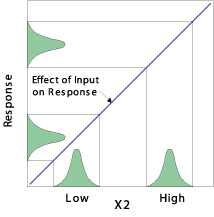

Obviously, reducing response variation is a highly desirable outcome. But what if the absolute level of response gets shifted out of specification? Ideally, you will find another control factor that affects response in a linear fashion, such as that shown in Figure 3. (We added the bell-shaped curves to indicate that variation will likely be distributed according to a normal distribution.)
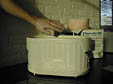
Let's see how these concepts can be applied to a six-sigma project on a coatings system.

A Case Study on POE:
Powder Coating a Metal Part
The Therabath paraffin therapy bath5 is a durable medical device that holds one gallon of molten paraffin wax. Sufferers of osteoarthritis use it for physical therapy. They dip their hands repeatedly in the heated bath, which helps loosen stiff joints. The wax then slowly solidifies as a glove, producing further therapeutic benefits through the heat of fusion. Oils reduce the overall melt point to a comfortable level, facilitate removal of the glove and provide moisturizing for skin.The tank holding the molten wax is made out of stamped steel. It's then powder coated electrostatically with an epoxy-based paint. The coating must withstand temperatures approaching 130 degF. and exposure to salt-water that collects from the sweat of the Therabath users.
The units are sold with a life-time guarantee. Very few units get returned, but in those that do, two types of off-grade predominate.
- Blisters that appear spontaneously after some time in use
- Scratches that become noticeably corroded.

Designing An Experiment That Combines Mixture with Process Variables
Our design of experiments combined variations in mixture components and process factors. (See our previous article1 for details on "crossed" designs like this.) Table 1 shows the names and ranges of the input variables, as well as their expected variation in terms of standard deviation, which will come into play when calculating POE.
The mixture variables (A, B and C) were added to a constant of 90 weight % of the powder. The other 10% was made up of bisphenol A, aluminum oxide and silica, all held in constant proportion. The paint chemists expected the three main powder-coating components to interact in complex ways. To properly model such behavior, we wanted to formulate a sufficient number of unique blends to fit a "special cubic" mixture model:


These two models were crossed to account for possible interactions between mixture and process. For example, the ideal coating formulation may differ depending on the choice of bake time and temperature, which could be a function of the particular type oven available for processing the tanks. The crossed model contains 42 terms (7 from the special cubic mixture model multiplied by 6 from the quadratic process model). With the aid of computer software,6 a d-optimal design (described in a previous article2) was generated to provide the ideal combinations of variables for fitting response data to the combined mixture-process model. We added five extra unique points for estimating lack-of-fit for the model, plus five replicates of existing points to estimate pure error. We also incorporated one center point (combination #3 on the list), representing the standard operating conditions, for a grand total of 53 combinations.
The results (simulated) of this experiment can be seen in the Appendix. The combinations are organized for easier viewing, with replicate points lumped together. However, experiments like this should always be performed as randomly as possible to offset any lurking variables, such as ambient humidity.

Generating 3D Surfaces to See Impact of Inputs on Responses and POE
Without computer software to do the number-crunching, it would be incredibly difficult to fit all the coefficients in the mixture-process crossed models. It's very cumbersome to even sort through all the statistics generated by such software. We advise that you make use of computerized tools for reducing models to their bare essentials. Make sure that the results pass your pre-set thresholds for statistical significance (typically 95% confidence) and that you don't violate any of the standard assumptions for normality (diagnostic plots help you check this). In this case, the statistical analysis produced highly significant models that met all assumptions. To assess the outcome of an experiment like this, one picture is worth a thousand numbers, so let's not get bogged down in the statistics. Instead, take a look at the response surfaces for the mixture variables (see Figure 6a and 6b) and the process factors (see Figure 7a and 7b) for adhesion and hardness.


Finding a Setup that Meets All Specifications with Minimum POE
As noted earlier, the goals for the Therabath powder-coating properties are an adhesion of at least 200 and a hardness of 140, achieved on a consistent basis by minimizing POE. Furthermore, it would be nice for bake time to be minimized, thus increasing throughput. Obviously, to accomplish all this, some tradeoffs must be made. Therefore, it's necessary to allow for some ranges on all of these goals. Table 2 shows what the Therabath engineers are willing to accept.
The input variables aren't listed in the table, but they do get constrained within their previously specified ranges. Never extrapolate outside of your experimental region because the predictive models probably won't work out there. Stay in bounds or possibly face some dangerous consequences!

Two "sweet spots" can be seen in the process space (bake temperature versus bake time) by overlaying contour plots for hardness and adhesion, with unacceptable regions shaded out (see Figure 9). (The formulation of the powder-coat is set at the optimal component levels shown in Table 3.)

At the values of 15 minutes for bake time at 364 deg (approximately what's shown in the lower flag in Figure 9) the POE of adhesion exceeds 23 units of standard deviation, a substantial increase from the 18.9 result reported in Table 3 for the optimum setup. In companies that place an emphasis on six-sigma goals this may be an unacceptable trade-off. However, if time is of the essence, it's easy enough to incorporate this in the optimization criteria - simply add a goal to minimize it. That's what makes the whole DOE procedure outlined in this article so incredibly powerful: It produces statistically valid predictive models that can be easily manipulated for "what-if" analysis. Then when goals shift, or get weighted differently, it's just a matter of plug-and-chug and the answer magically appears. To ensure that it's correct, confirmation runs must be performed. Hopefully, the results will agree well with what's predicted to happen.
Conclusion
As a result of doing systematic experimentation, using sound statistical principles, the quality of the powder-coating of Therabath tanks can be improved and became more robust to variations in the levels of components and processing factors. With the proper knowledge and software tools, coatings chemists and engineers can apply these same methods to their systems and accomplish similar breakthrough improvements.For more information on design of experiments, contact Stat-Ease Inc., 2021 East Hennepin Ave., Suite 191, Minneapolis, MN 55413; phone 612/378.9449; fax 612/378.2152; e-mail Mark@StatEase.com; or Circle Number 80.
References
1 Anderson, M.J.; Whitcomb, P.J. "Design Experiments that Combine Mixture Components with Process Factors," PCI November 2000.2 Anderson, M.J.; Whitcomb, P.J. "Computer-Aided Tools for Optimal Mixture Design," PCI, November 1999.
3 "Making Six Sigma Benefits Stick," Chemical Week Vol. 163, No. 19. p. 40, 5/9/2001.
4 Phelan, S. "Six Sigma, "Information Technology & You: Creating A Happy Customer," PCI, website http://www.pcimag.com/pci/cda/articleinformation/features/bnp__features__item/0,1846,388,00.html
5 WR Medical Electronics Co., 123 N. 2nd St., Stillwater, MN 55082.
6 Helseth, et al, Design-Expert, Version 6 for Windows, Stat-Ease Inc., Minneapolis, 2001.
7 Anderson, M.J.; Whitcomb, P.J. "Optimization of Paint Formulations Made Easy with Computer-Aided Design of Experiments for Mixtures," J. Coatings Technol., Volume 68, Number 858, July 1996, pages 71-75.
Looking for a reprint of this article?
From high-res PDFs to custom plaques, order your copy today!